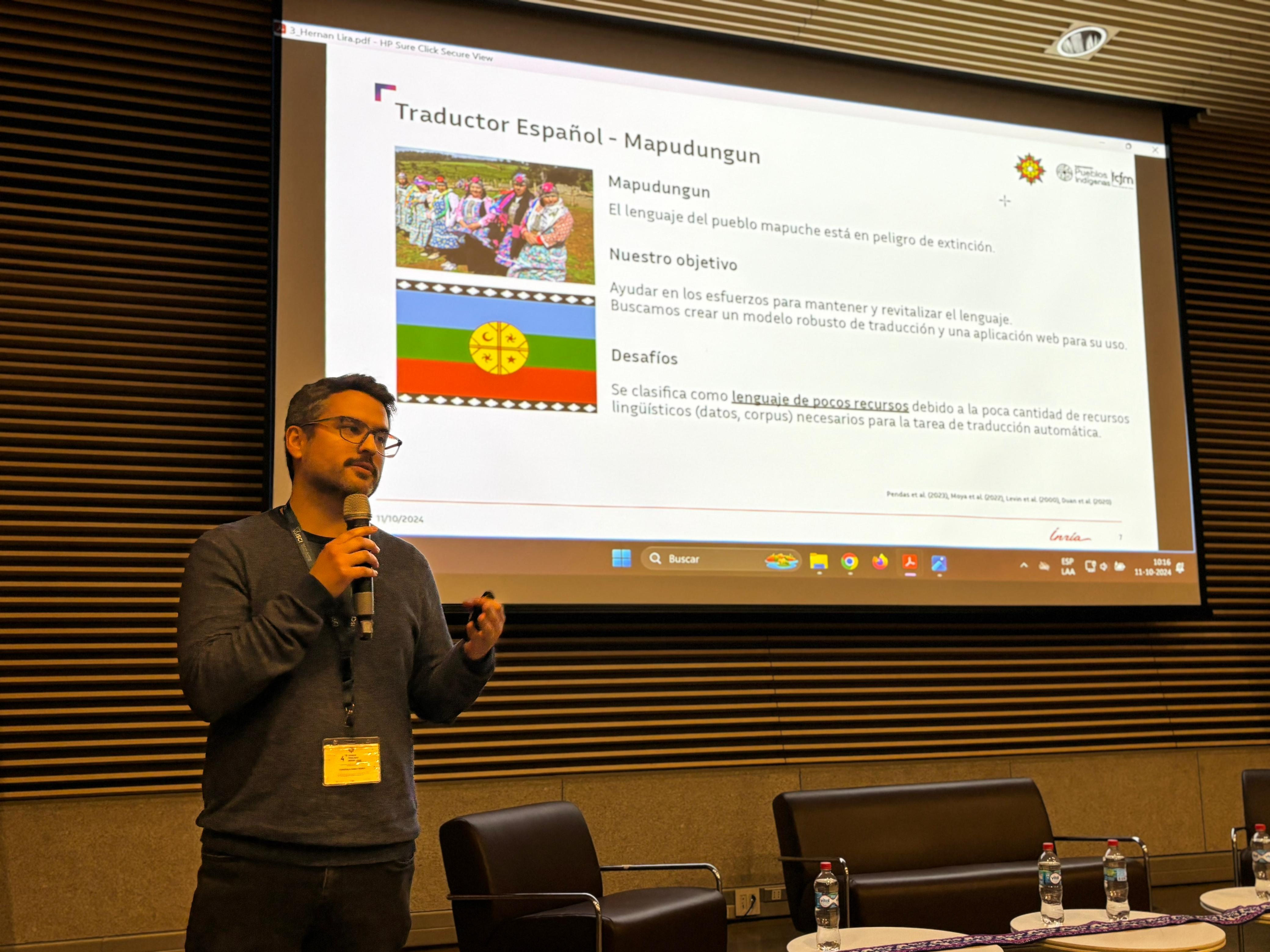
During Inria Chile's participation in the latest edition of the Indigenous Technology Congress (Congreso Tecnológico Indígena), an important advance of the project that seeks to translate automatically from Mapudungún to Spanish, called Human-AI Ensembled Machine Translation for Underrepresented Languages (Huemul), was revealed.
Huemul, developed by Inria Chile and led by Nayat Sánchez-Pi, Director of Inria Chile and Luis Martí, Scientific Director of Inria Chile, was presented at the Congress by Hernán Lira, AI Researcher & Data Scientist at Inria Chile, explaining that the project seeks to contribute to the preservation of Mapudungún.
One of the particularities of the initiative is that Mapuche communities were included in the development and validation of the model, which has allowed the inclusion of vulnerable groups in AI issues, not only benefiting indigenous communities, but also making them co-creators of technology.
Verbatim
The development of artificial intelligence has been strongly influenced by the dominance of English-speaking countries, particularly the United States and the United Kingdom. This dominance has led to a significant underrepresentation of many languages in AI research and development. AI systems trained on predominantly English language data may exhibit cultural biases that are not relevant to other languages and cultures. This is the case of our project that contributes to the preservation of the cultural heritage of Indigenous and Native Peoples, as is the case of languages such as Mapudungun.
Director of Inria Chile
The efforts to preserve a language at risk
One of the main difficulties of the project is that Mapudungún has historically been transmitted orally and with very little written support in digital format. There is also a key concept in this project, which is the “corpus”, a set of texts that is used to train AI systems. To create an efficient translator, a dataset of Spanish phrases along with Mapudungun and their translations is required.
However, Mapudungun has little digitized documentation, making it difficult to form a comprehensive and standardized corpus. In addition, its morphological complexity-being polysynthetic and agglutinative-further complicates the task of AI models to perform accurate translations.
Even so, Inria Chile's Huemul project has opted for an approach based on neural networks to overcome these challenges. These networks, which are capable of learning patterns by analyzing large amounts of data, allow AI models to “understand” the languages they work with. In this case, a technique called transfer learning is used, where an AI model is initially trained with a large corpus of a resource-rich language, such as Spanish and English, and then adjusted to work with resource-poor languages, such as Mapudungun. This approach reduces the need for large volumes of Mapudungun data, which is essential given the scarcity of Mapudungun texts and its linguistic complexities, given its polysynthetic and agglutinative structures.
The experiments included a large corpus of 260,000 sentences derived from Mapudungun conversations, where the researchers tested different configurations to understand how to improve the translations. Questions such as: Does the target language of the pre-trained model (in this case English) need to have similar characteristics to Mapudungun for the model to work better? To answer this, models trained with binding languages such as Finnish and others with non-binding languages such as English were compared.
Results and access to the project
The preliminary results of the project are encouraging, suggesting that transfer learning is a powerful tool for improving the quality of translation between Spanish and Mapudungún, and that structural similarity between languages is not a determining factor in this case, as well as a significant improvement in the quality of translations using the transfer learning approach.
As part of the project, a web application has been developed that allows users to enter text in Spanish to obtain its translation into Mapudungún and is available free of charge on the Hugging Face platform.