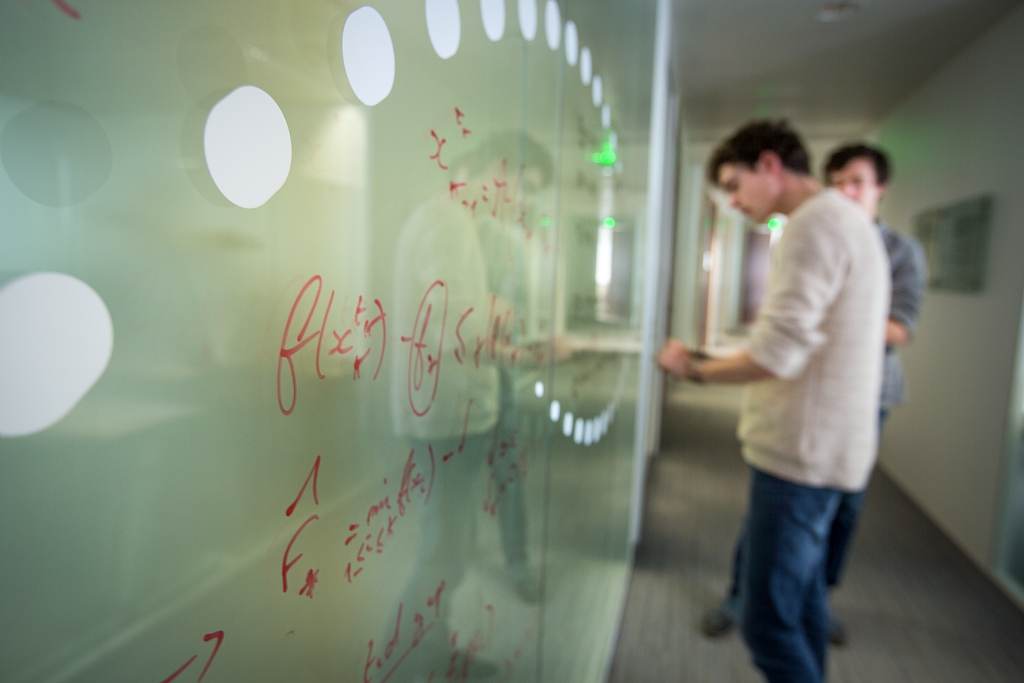
In an increasingly digitized world, the French-Chilean Associate Team, where researchers from the Inria Paris Centre, Sierra and the Pontificia Universidad Católica de Chile (PUC) collaborate, has been engaged in research on first-order accelerated methods for machine learning. This includes a range of disciplines, such as optimal convergence in statistical learning, stochastic convex optimization, variational inequalities and fixed point problems under performance estimation. The goal is to achieve applications and push the boundaries in multiple areas, such as astronomical data analysis, seriation problems, signal processing, engineering and training of generative adversarial models (GANs).
This time, we spoke with Alexandre d'Aspremont, from the SIERRA project-team and researcher and coordinator of FOAM, and Cristóbal Guzmán, researcher and professor specialized in large-scale convex optimization, complexity of iterative methods, equilibrium in transport/telecommunications networks at PUC, one of the best universities in Latin America and Chile, to learn more about their collaborative work.
Could you present FOAM to us your?
FOAM (First-Order Accelerated Methods for Machine Learning) is an associate team based on the collaboration between the INRIA Sierra team and researchers from the Pontificia Universidad Católica de Chile and Universidad Adolfo Ibañez. The co-PI from the French side is Alexandre d’Aspremont, and the co-PI from the Chilean side is Cristóbal Guzmán.
About the origin of FOAM, how did you started to collaborate in the first time?
- Cristóbal Guzmán (C. G.): I started collaborating with Alex as early as in my PhD. We had common interests in the complexity of optimization, which lead to a long-term collaboration. The main motivation for the associate team was as a vehicle to facilitate the involvement of students and other researchers on this long-term project.
- Alexandre d’Aspremont (A. A.): We had started collaborating on several topics of common interest as early as 2013 around the complexity of first-order methods. This was followed by several visits in both Paris and Chile. We also co-supervise a PhD student and the associated team format seemed very appropriate to support this ongoing collaboration.
Which are the specific areas of research of each of you? and what scientific questions are you seeking to answer or have already answered with the project?
- C.G.: I am interested in the complexity of optimization, especially in connection to solving machine learning problems. Relatedly, I have worked recently on these questions in settings involving private user data; here the main challenge is designing algorithms with rigorous privacy guarantees. This project has been instrumental to advance understanding in these fields, and furthermore we have explored some machine learning problems which go beyond more traditional optimization, such as seriation problems.
- A. A.: My work mostly focuses on understanding algorithmic complexity and performance. The idea is to identify regularity properties of optimization problems that better predict their complexity and more closely align with empirical evidence. The idea is to better understand the theoretical limits of current methods and improve their performance in practice.
What are the objectives and expected results of FOAM?
- C. G.: Our objectives can be summarized in developing a fundamental understanding for solving various models recently popularized in machine learning, including stochastic optimization, variational inequalities and fixed point problems. This research is complemented by the development of efficient methods to solve this problems. Expected results of the project are publications in top notch conferences and journals which focus on these areas of research. We also expect to graduate a significant number of MSc and PhD students at the involved institutions.
What are the specific applications that FOAM could have or has had?
- C. G.: Our main inspiration for the theory and methods we are developing is related to inverse problems. This is a setting where there is an unknown signal (this could be audio, image, etc.) from which one only observes it partially (e.g., by passing through a filter or mask), and then the goal is to accurately reconstruct it.
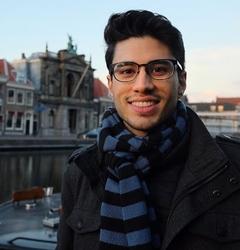
Verbatim
The goal is to accurately reconstruct that signal, while in general performing such reconstruction is not possible, exploiting the structure of the signal (e.g., that it is simple enough) one can embed this prior information into an optimization model. With very large models, the efficiency of solving this optimization model becomes paramount, and that’s where our research contributes to.
Assistant Professor, Pontificia Universidad Católica de Chile
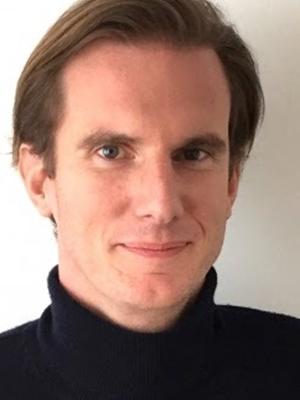
Verbatim
Optimization is used in a very broad array of disciplines, ranging from signal processing, machine learning, engineering to bioinformatics. We hope our methods will push the boundaries on what can be achieved in these fields, both in terms of scale and performance.
Researcher, Inria SIERRA project-team
How is the work between the French and Chilean teams complementary?
- C. G.: I believe the members from France and Chile have a highly complementary nature. On the one hand, SIERRA has a very strong record on computational methods and involvement in applications. Aside from that, they are highly active in the machine learning community. On the other hand, the Chilean members of the team have a more theoretical focus. I believe that these complementary perspectives have been key to maintain a healthy balance of theoretical and computational focus on our research. Namely, the theoretical work we conduct is heavily rooted on developing methods that can be readily used in other settings.
INRIA funding has been crucial to my career development. It has boosted and expanded my research, facilitated my connections with the French researchers, and it has provided opportunities for young researchers in both groups.
- A. A.: On the French side, we have been working heavily on improving complexity bounds and algorithmic performance, while the Chielan team has a deep expertise on the theoretical results telling us how far we can expect to go in this vein, i.e. theoretical lower bounds on this performance.
With the covid episode finally over, it’s great to be able to restart in person collaborations, especially long distance ones. The FOAM project is a great opportunity to do so.
¿What is an Associate Team?
An associate team is a joint research project between an Inria project-team and a foreign research team. For a period of 3 years, the partners jointly define a scientific objective, a research plan and a program of bilateral exchanges.
Since Inria's arrival in Chile in 2012, 29 French-Chilean research projects in different areas of digital sciences have been funded under this program by Inria.
Currently, there are nine Associate Teams working, in which researchers from Inria centers in France collaborate, such as the Inria Centre at the University of Bordeaux, Inria Centre at the University of Grenoble Alpes, Inria Centre at the University of Lille, Inria Lyon Centre, Inria Nancy - Grand Est Centre, Inria Paris Centre, Inria Centre at Rennes University, Inria Antenna at the University of Montpellier, Inria Saclay Centre, Inria Centre at Université Côte d’Azur; and Chilean institutions, such as Universidad de Chile, Pontificia Universidad Católica de Chile, Universidad del Bío Bío, Pontificia Universidad Católica de Valparaíso, Universidad Adolfo Ibáñez and Universidad de O'Higgins.