- Thursday, January 30, 2025 - 11:00 am (Santiago de Chile time)
- Speaker: Stéphanie Chaillat is a CNRS Research Director at POEMS, a joint CNRS-INRIA-ENSTA Paris team. She received her PhD in computational mechanics from École Polytechnique in 2008 and later completed a postdoctoral position at Georgia Tech's College of Computing before joining CNRS in 2010.
- Hybrid format
- The talk will be in English
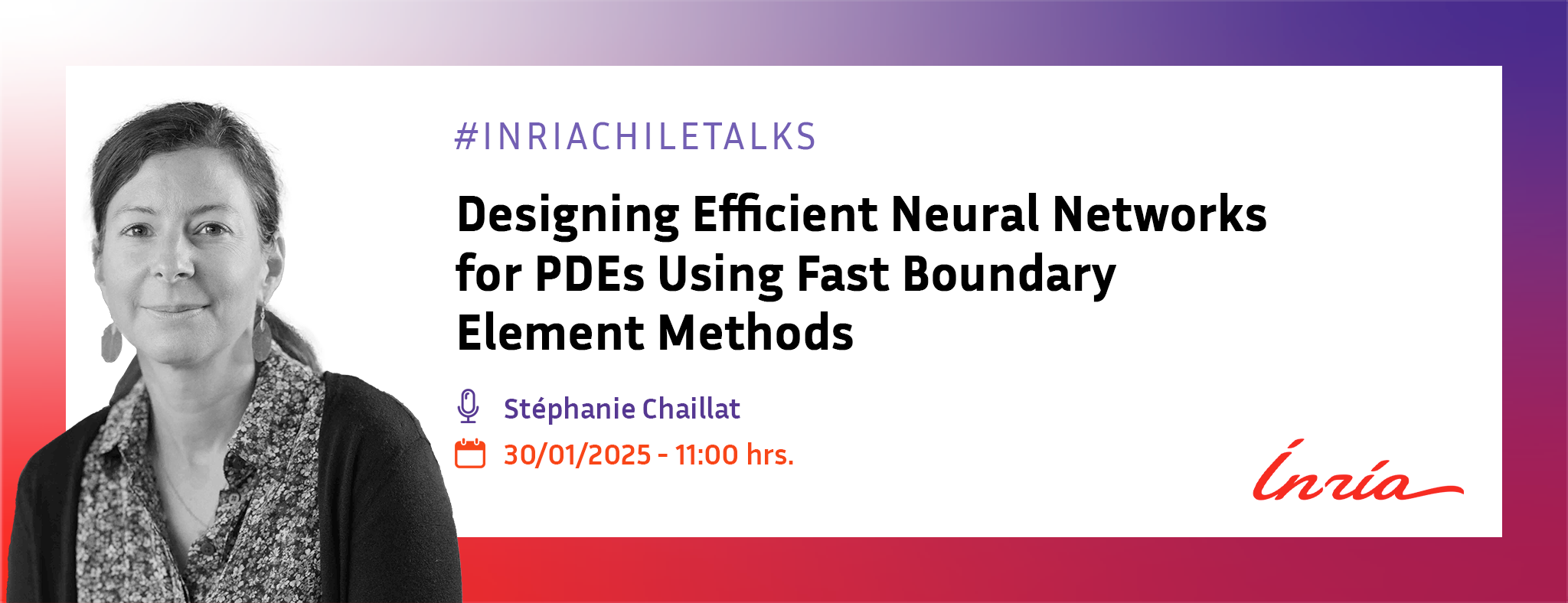
Abstract
Gas-filled bubbles are crucial in many scientific and engineering fields, with applications ranging from medical therapies to geophysical explorations. Simulating their dynamics, particularly the complex expansion and collapse phases, is challenging due to the need for high accuracy over evolving geometries and multiple oscillation cycles. Traditional methods, like the boundary element method (BEM), solve exterior Laplace problems at each time step but are computationally intensive and prone to numerical instabilities.
In this work, we leverage our expertise in fast BEMs to design specialized graph neural networks (GNNs) tailored for dynamic geometries. Unlike standard GNNs, which often suffer from oversmoothing, our approach incorporates BEM-inspired insights to maintain sharp representations and ensure computational efficiency. This method offers a promising path to accelerate simulations while preserving accuracy and stability.
This is a joint work with Ian McBrearty from Stanford University.
Stéphanie Chaillat
Stéphanie Chaillat is a CNRS Research Director at POEMS, a joint CNRS-INRIA-ENSTA Paris team. She received her PhD in computational mechanics from École Polytechnique in 2008 and later completed a postdoctoral position at Georgia Tech's College of Computing before joining CNRS in 2010.
Recently returned from an extended research stay at Stanford University's Department of Geophysics, where she explored neural network methodologies, her primary research focuses on designing fast and efficient algorithms to simulate realistic wave propagation phenomena, with a particular emphasis on boundary element methods (BEMs).
This presentation will connect her foundational work on BEMs with the insights she gained into neural networks, demonstrating how these approaches can complement each other to address complex wave simulation challenges.